Single digit APCER decrease
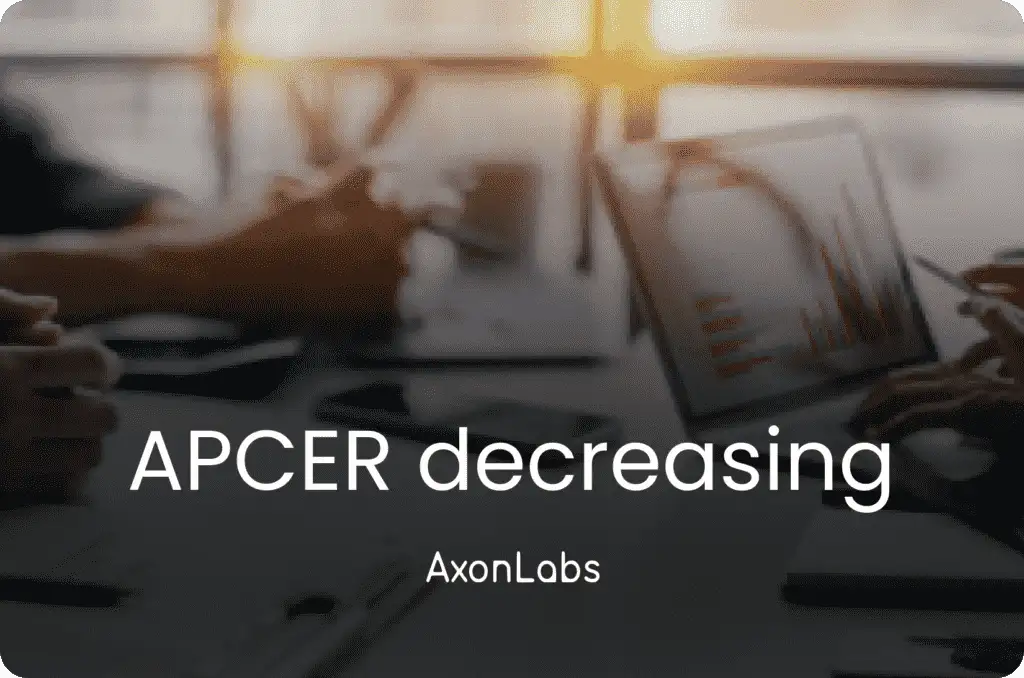
Task
A Head of sourcing and Computer vision software engineer from a European e-KYC startup approached us. They were facing some challenges with their liveness detection model. Although the model had a lot of production data, it could still be affected by some of the most common spoofing attacks, such as print attacks, cut attacks, and replay attacks. The client required a solution to enhance the model’s accuracy and ensure robust performance in real-world scenarios
Solution
The client’s existing production data was insufficient due to a lack of genuine individual selfies. We proposed developing a customized dataset specifically tailored to their needs, to enhance their liveness detection technology.
Our Solution Included:
- Relevant Dataset: We delivered a dataset containing both videos with targeted movements and authentic selfies for each individual, improving the liveness detection capabilities
- Targeted Training: The dataset focused on the most prevalent spoofing techniques (print, cut, and replay), ensuring the model could effectively mitigate these threats
- High-Quality Data: The dataset was meticulously curated to encompass a broad spectrum of real-world conditions, guaranteeing the model’s resilience against diverse anti-spoofing challenges
Result
Reduced Error Rate: The model’s Attack Presentation Classification Error Rate (APCER) decreased by a single-digit percentage, significantly enhancing the accuracy of liveness detection
Improved Generalization: The customized dataset, featuring paired videos with targeted movements and authentic selfies, enabled the model to better generalize and distinguish between real and spoofed attempts
Enhanced Security: By focusing on the most prevalent attack types (print, cut, and replay), the model became stronger against real-world anti-spoofing challenges, ensuring a more secure e-KYC process